My Academic Contribution
Throughout my career, I have actively engaged in academic discourse and sought to make meaningful contributions across three key themes. These include developing quantitative methods to map scientific discourse, enhancing the measurement of science and technology emergence, and analyzing the impact of technology (i.e. artificial intelligence) on business productivity. Read more under each contribution cluster.
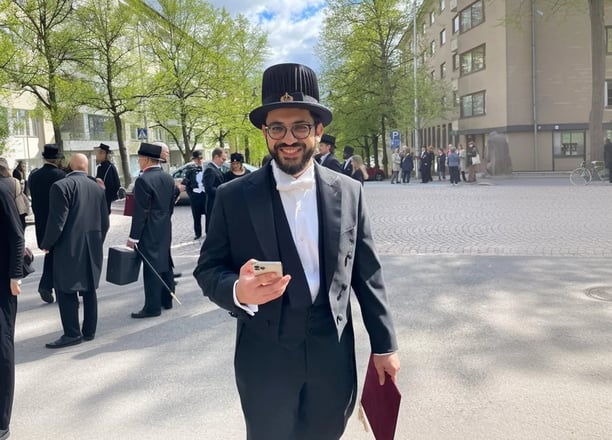
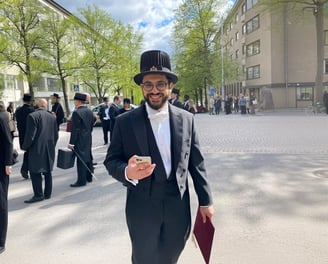
2.Measuring Innovation and Technological Emergence
This theme emphasizes enhancing methods for tracking the emergence of science and technology and their alignment with broader societal goals. It includes creating models and tools that identify patterns and trends in innovation, offering actionable insights for policymakers and researchers.
3.Technology Role in Business Transformation
This theme explores the transformative impact of artificial intelligence on business productivity and innovation. It examines AI adoption strategies, their effects on organizational performance, and the integration of AI-driven tools to optimize processes and promote sustainable growth.
1.Mapping Scientific Progress
This theme focuses on developing quantitative frameworks to systematically analyze and map the evolution of scientific concepts and technologies. By utilizing advanced bibliometric techniques and natural language processing, it provides valuable insights into the trajectory of scientific research and innovation.
1.Mapping Scientific Progress
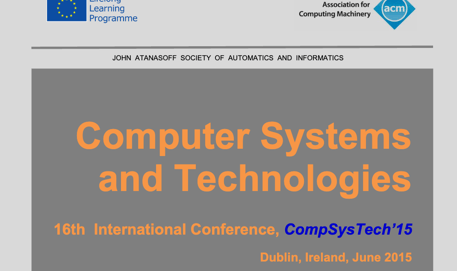
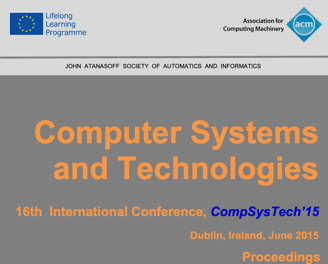
This paper addresses the challenge of tracking the growing volume of scientific publications by introducing an open, cloud-based solution for bibliographic analysis. The tool automates time-consuming steps in systematic mapping studies, providing a more accessible and efficient method for researchers to analyze the state of the art in various scientific fields.
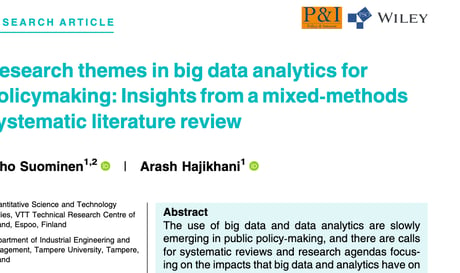
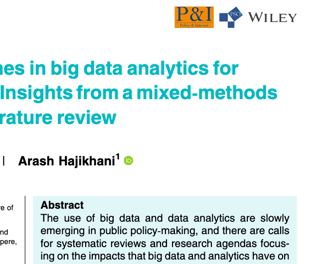
This paper investigates the emerging role of big data and data analytics in public policy by conducting a literature review using bibliometric and qualitative analyses. It identifies nine key thematic clusters, with a focus on big data's impact on the policy cycle, data-driven decision-making, and productivity, and proposes a research agenda to guide future studies in this field.
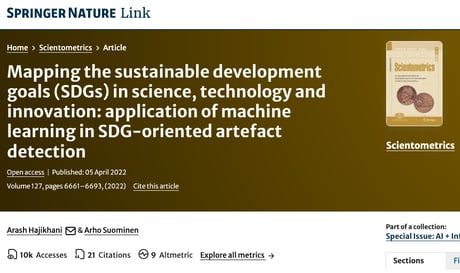
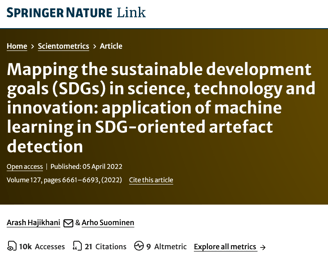
This paper explores the alignment of science and technology with Sustainable Development Goals (SDGs) by developing a machine learning model to classify SDG relevance in patent documents. The findings highlight the extent to which patents address SDGs, offering insights to improve the alignment of R&D efforts, patenting strategies, and contributions toward achieving global sustainability goals.
2. Measuring Innovation and Technological Emergence
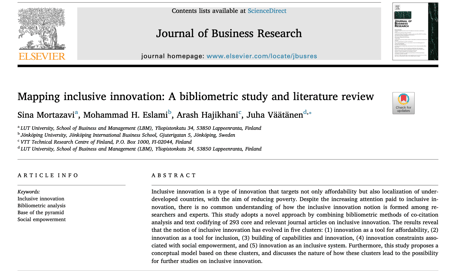
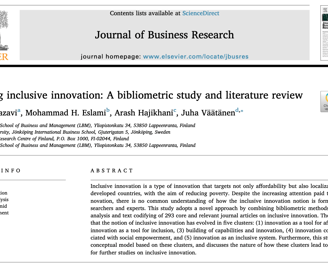
The paper explores the evolving concept of inclusive innovation, which focuses on affordability and localization to address poverty in underdeveloped regions. Using bibliometric methods, the study identifies five key thematic clusters and proposes a conceptual model to guide future research on inclusive innovation and its role in fostering social empowerment and systemic inclusion.
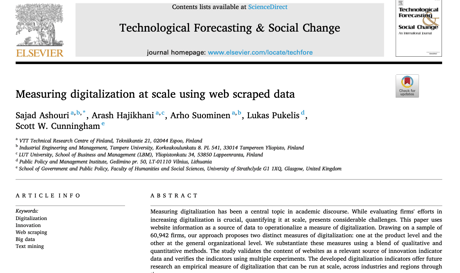
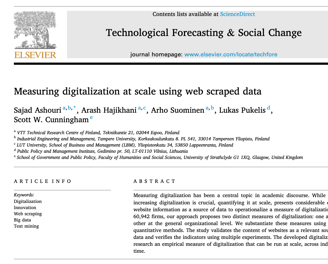
This paper investigates the productivity effects of AI adoption using job advertisement data and firm-level productivity metrics. The findings reveal that AI adoption increases productivity but primarily in large firms, with delayed benefits due to technological immaturity and a three-year investment lag, shedding light on the "AI-related return of the Solow paradox."
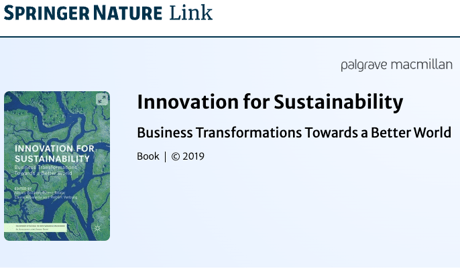
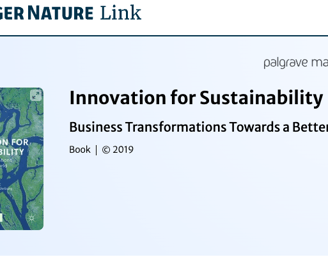
This book chapter presents a literature review and bibliometric analysis on innovation for sustainability, focusing on five goal-oriented discussions: strategic, operational, organizational, collaborative, and systemic. The study highlights the evolution of the subject across disciplines since 2002 and offers insights for researchers and practitioners to bridge scientific knowledge with practical applications in organizations and businesses.
3.Technology Role in Business Transformation
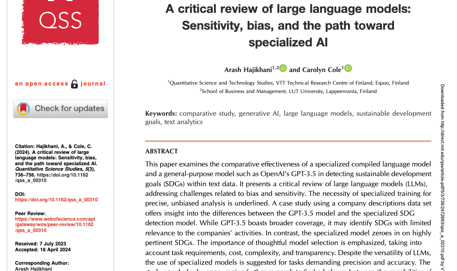
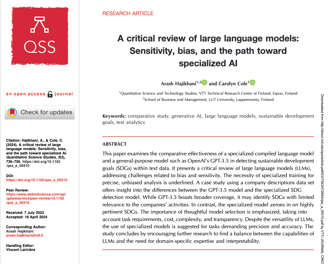
This article critically reviews large language models (LLMs), highlighting their sensitivity, bias, and limitations in analyzing text data. It emphasizes the importance of specialized models for precise and unbiased analysis, demonstrating their advantages over general-purpose models in tasks requiring domain-specific accuracy and relevance.
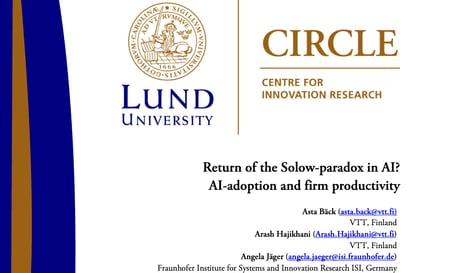
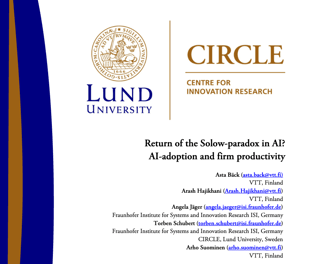
This paper investigates the productivity effects of AI adoption using job advertisement data and firm-level productivity metrics. The findings reveal that AI adoption increases productivity but primarily in large firms, with delayed benefits due to technological immaturity and a three-year investment lag, shedding light on the "AI-related return of the Solow paradox."
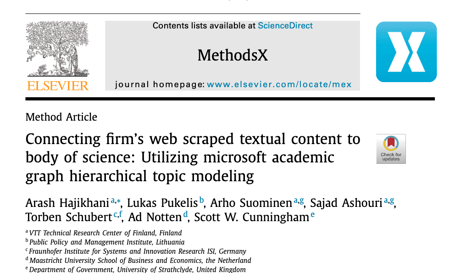
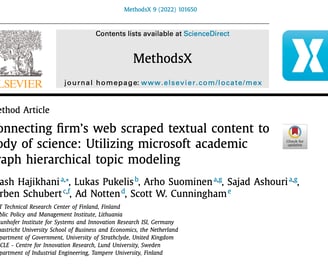
This paper presents a method to link textual data from company websites to scientific knowledge using Natural Language Processing (NLP). By connecting economic classification systems like NACE with Microsoft Academic Graph topic modeling, the study demonstrates how web scraping, NLP, and data transformation can bridge traditional classifications with insights from new data sources.
Arash Hajikhani, PhD
Showcasing my academic profile and projects and blogpost series
contact
Blogpost Subscription
© 2024. All rights reserved.